How to learn Generative AI models in 2025
How to learn Generative AI
Generative AI (GenAI) is transforming industries by enabling machines to create text, images, audio, code, and more. For a graduate student eager to learn GenAI, it’s essential to build a solid understanding of the underlying models, tools, and techniques. This tutorial will guide you through a structured learning approach, leveraging key models mentioned earlier.
1. Fundamentals of Generative AI
What is Generative AI?
Generative AI is a type of artificial intelligence focused on creating new content, such as text, images, audio, video, and even code, by learning patterns from existing data. Unlike traditional AI, which is designed to analyze data and make predictions, generative AI aims to produce original outputs that mimic human creativity.
How Does Generative AI Work?
Generative AI models are typically powered by advanced machine learning algorithms, particularly deep learning. These models learn from large datasets to understand patterns, relationships, and structures, enabling them to generate novel content. Key techniques include:
Prerequisites
Before diving into GenAI, ensure you have:
-
Mathematics: Linear algebra, probability, and calculus.
-
Programming Skills: Python is essential.
-
Machine Learning Basics: Familiarity with neural networks, backpropagation, and optimization.
-
Deep Learning Frameworks: PyTorch or TensorFlow.
2. Learn Transformer-Based Models
Transformers are the backbone of many generative AI systems.
Step 1: Understand Transformers
-
Read Vaswani et al.'s paper “Attention is All You Need”.
-
Implement a basic transformer from scratch using PyTorch/TensorFlow.
-
Tools: Hugging Face Transformers library.
Step 2: Explore GPT Models
-
GPT-3/4:
-
Learn their architecture and capabilities.
-
Experiment with OpenAI’s API to build applications like chatbots.
-
-
T5:
-
Study text-to-text generation using T5.
-
Practice on datasets like summarization or translation tasks.
-
Step 3: Dive into BERT Variants
-
Understand how models like RoBERTa and DistilBERT improve efficiency.
-
Use them for embedding generation or pretraining tasks.
3. Dive into Diffusion Models
Diffusion models power text-to-image systems like DALL·E and Stable Diffusion.
Step 1: Understand Diffusion Processes
-
Study the theory of denoising diffusion probabilistic models (DDPMs).
-
Implement a basic diffusion model.
Step 2: Work with Stable Diffusion
-
Install and use Stable Diffusion for image generation.
-
Experiment with prompts and fine-tune the model for custom tasks.
Step 3: Experiment with Imagen
-
Review Google’s Imagen for generating photorealistic images.
-
Use pretrained models for text-to-image synthesis.
4. Explore GANs (Generative Adversarial Networks)
GANs are foundational for image generation.
Step 1: Learn GAN Basics
-
Read Goodfellow et al.’s paper introducing GANs.
-
Implement a simple GAN for MNIST digit generation.
Step 2: Study Advanced GANs
-
StyleGAN: Learn to create high-quality images like human faces.
-
CycleGAN: Practice image-to-image translation tasks (e.g., photo to painting).
Step 3: Compare with Diffusion Models
-
Understand how diffusion models outperform GANs in certain domains.
5. Multimodal Models
Multimodal AI combines text, images, and other data types.
Step 1: CLIP
-
Use OpenAI’s CLIP for connecting text and image embeddings.
-
Experiment with prompt engineering.
Step 2: Gato and Flamingo
-
Study DeepMind’s work for general-purpose multimodal tasks.
-
Explore applications combining text, image, and action.
6. Specialized Models for Specific Domains
Step 1: Code Generation
-
Use OpenAI’s Codex for programming tasks.
-
Build applications like GitHub Copilot.
Step 2: Speech and Audio
-
Work with WaveNet for speech synthesis.
-
Experiment with MusicLM for text-to-music generation.
Step 3: Large Multilingual Models
-
Explore BLOOM for multilingual tasks.
-
Practice translation and cross-lingual content creation.
7. Tools and Frameworks
-
Hugging Face: For NLP and multimodal tasks.
-
Stable Diffusion: For image generation.
-
LangChain: For building applications using LLMs.
-
Google Colab: For quick experiments.
-
Docker: For deploying models in production.
8. Build Projects
Capstone Ideas:
-
Chatbot: Build a GPT-based conversational agent.
-
Art Generator: Use Stable Diffusion or DALL·E for creating images.
-
Music Creator: Generate music using MusicLM.
-
Translator: Create a multilingual text generator using BLOOM.
9. Resources and Communities
-
Books: Deep Learning by Ian Goodfellow, Generative Deep Learning by David Foster.
-
Courses:
-
Coursera: Generative AI by DeepLearning.AI.
-
Udemy: Practical Generative AI with Python.
-
-
Communities:
-
Hugging Face forums.
-
Reddit: r/MachineLearning.
-
10. Stay Updated
Generative AI evolves rapidly. Follow:
-
Research papers on arXiv.
-
Blogs by OpenAI, Google AI, DeepMind.
-
Conferences: NeurIPS, CVPR, ICCV.
Learning Generative AI requires a mix of theoretical understanding, practical experimentation, and project development. By systematically approaching key models and tools, you can master the art of GenAI and build innovative applications that push the boundaries of creativity and technology.
Latest Posts
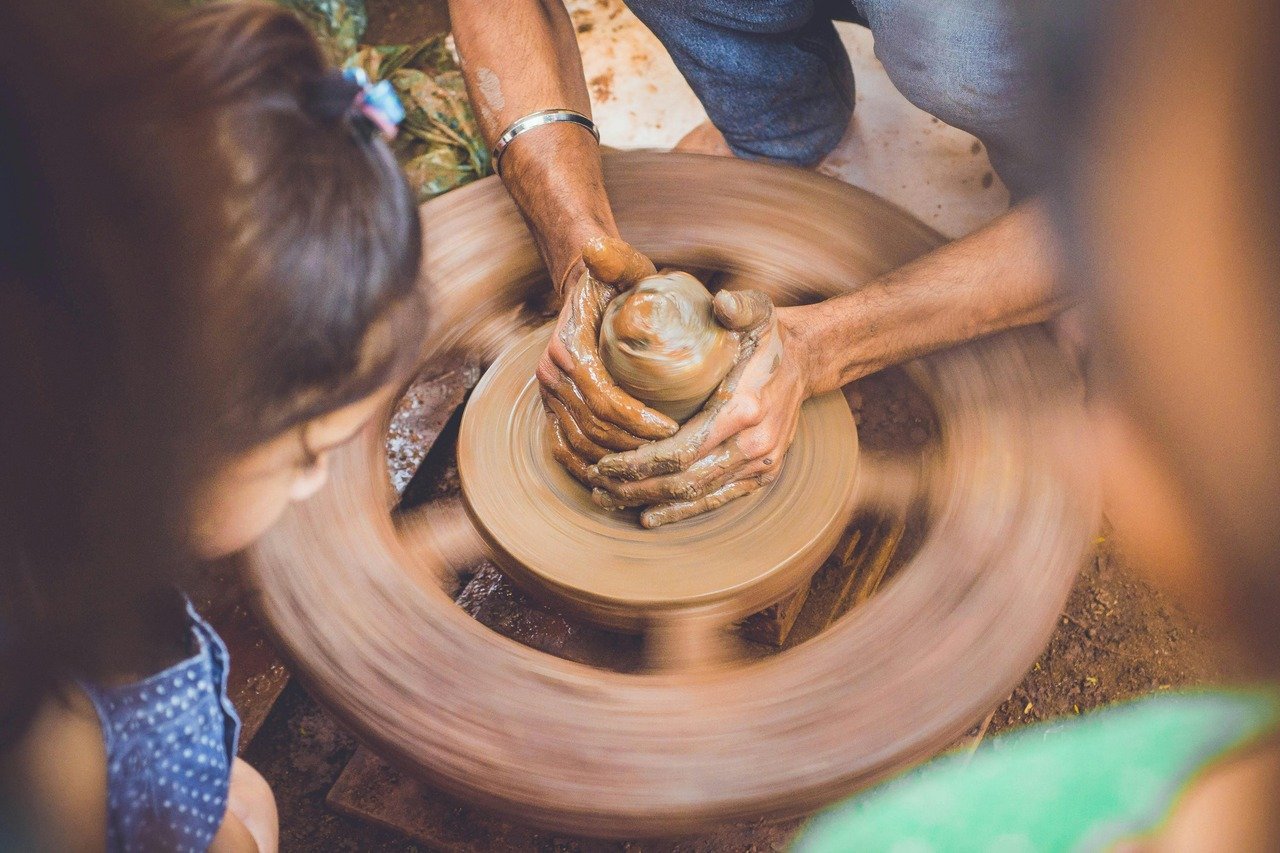
How do you manage ML experiments... Answer is MLFlow
MLflow is an open-source platform developed by Databricks to help manage the end-to-end machine learning lifecycle.
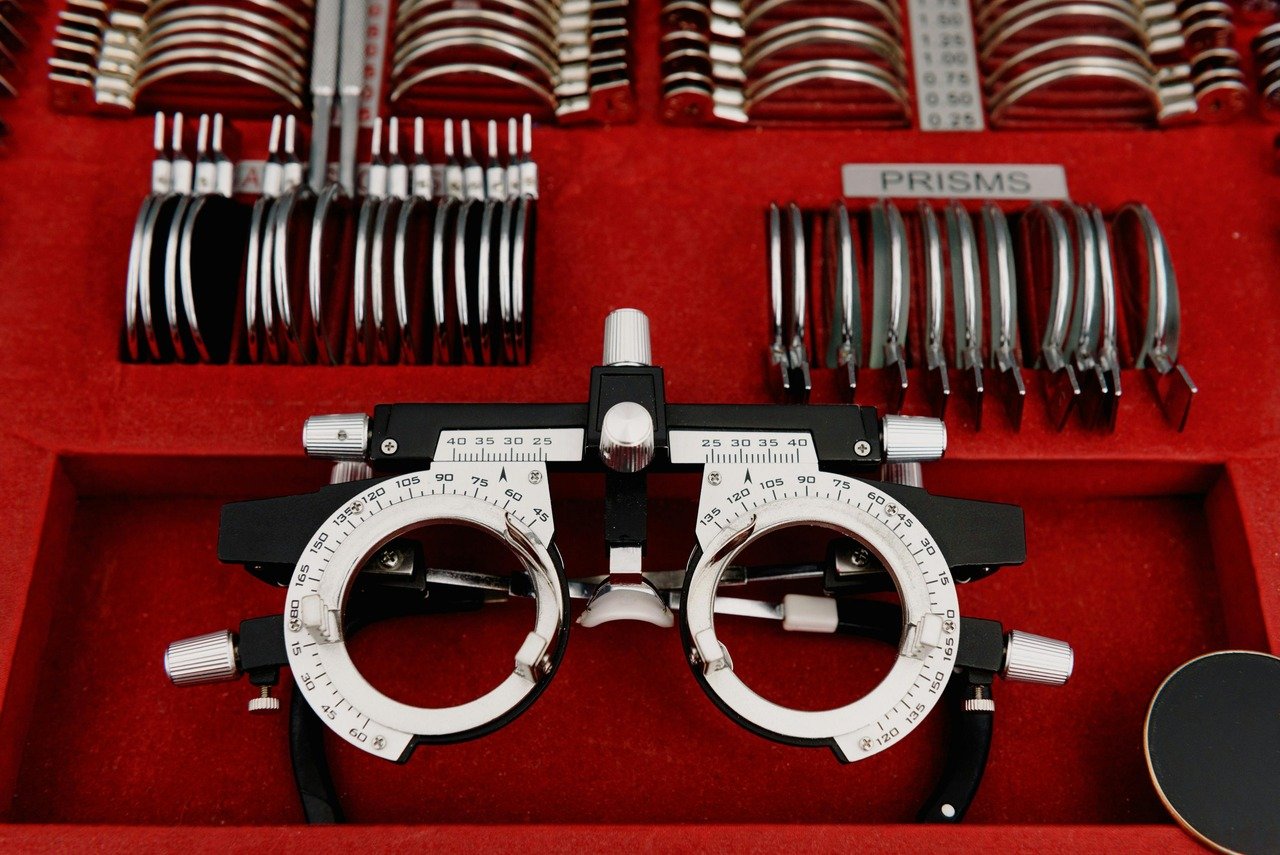
Brute Force Technique: Understanding and Implementing in JavaScript
Brute Force Technique: Understanding and Implementing in JavaScript