Introduction to Machine Learning
Introduction to Machine Learning
Machine learning (ML) is like teaching computers to think, learn, and adapt on their own. Imagine if your phone could predict what you want to text before you even type a word. How does that magic happen? Let’s break it down step-by-step.
1. What is Machine Learning?
Machine learning is a branch of artificial intelligence (AI) where systems learn from data instead of being explicitly programmed. Think of it as training a toddler. You don’t explain every detail but show them examples—over time, they figure things out.
But wait, here’s the twist:
Unlike toddlers, machines need rules to learn effectively. Without them, they’ll either overthink (overfit) or fail to understand patterns (underfit).
2. Why Does It Matter?
Ever wondered how Netflix knows what you’d like to watch next? Or how Amazon suggests products you didn’t know you wanted? It’s ML in action. By analyzing past data, machines can predict outcomes or classify objects in ways that improve with experience.
3. Types of Machine Learning
Understanding ML starts with its three primary types:
Supervised Learning:
It’s like a teacher-student relationship. The machine is fed labeled data (e.g., photos of dogs and cats labeled as such). Its job? Recognize patterns and make accurate predictions when faced with unseen data.
Unsupervised Learning:
Here, the machine has no teacher. It explores unlabeled data to find hidden patterns. Clustering customer segments or detecting anomalies in financial transactions are common uses.
Reinforcement Learning:
Think of a gamer earning rewards for making the right moves. Machines learn by trial and error, adjusting their approach based on feedback. This is how self-driving cars are trained.
4. How Does Machine Learning Work?
It’s all about data, algorithms, and training.
-
Collect Data:
Imagine teaching a machine to recognize cats. You’d start with hundreds (or thousands!) of images of cats.
Curious fact: The more diverse the data, the better the learning. -
Choose an Algorithm:
An algorithm is like a recipe. Some common ones include:- Linear Regression for predicting prices.
- Decision Trees for classification tasks.
- Neural Networks for complex patterns, like facial recognition.
-
Train the Model:
This is where the magic happens. The machine uses the data to adjust itself, learning patterns to improve its predictions. -
Test and Improve:
You test the model with new data. If it fails, you tweak it or get better data. Simple? Not always, but that’s the beauty of ML—it gets better with practice.
5. Challenges and Ethics
But here’s the catch:
Machines are only as good as their data. Biases in training data can lead to unfair or even dangerous decisions. That’s why ethical considerations in ML are more critical than ever.
Your First Steps
Want to try ML yourself? Start with Python—it’s beginner-friendly and has powerful libraries like Scikit-learn, Pandas, and TensorFlow. Begin by:
- Installing Python.
- Learning how to manipulate data.
- Exploring basic algorithms with tools like Google Colab (it’s free!).
Latest Posts
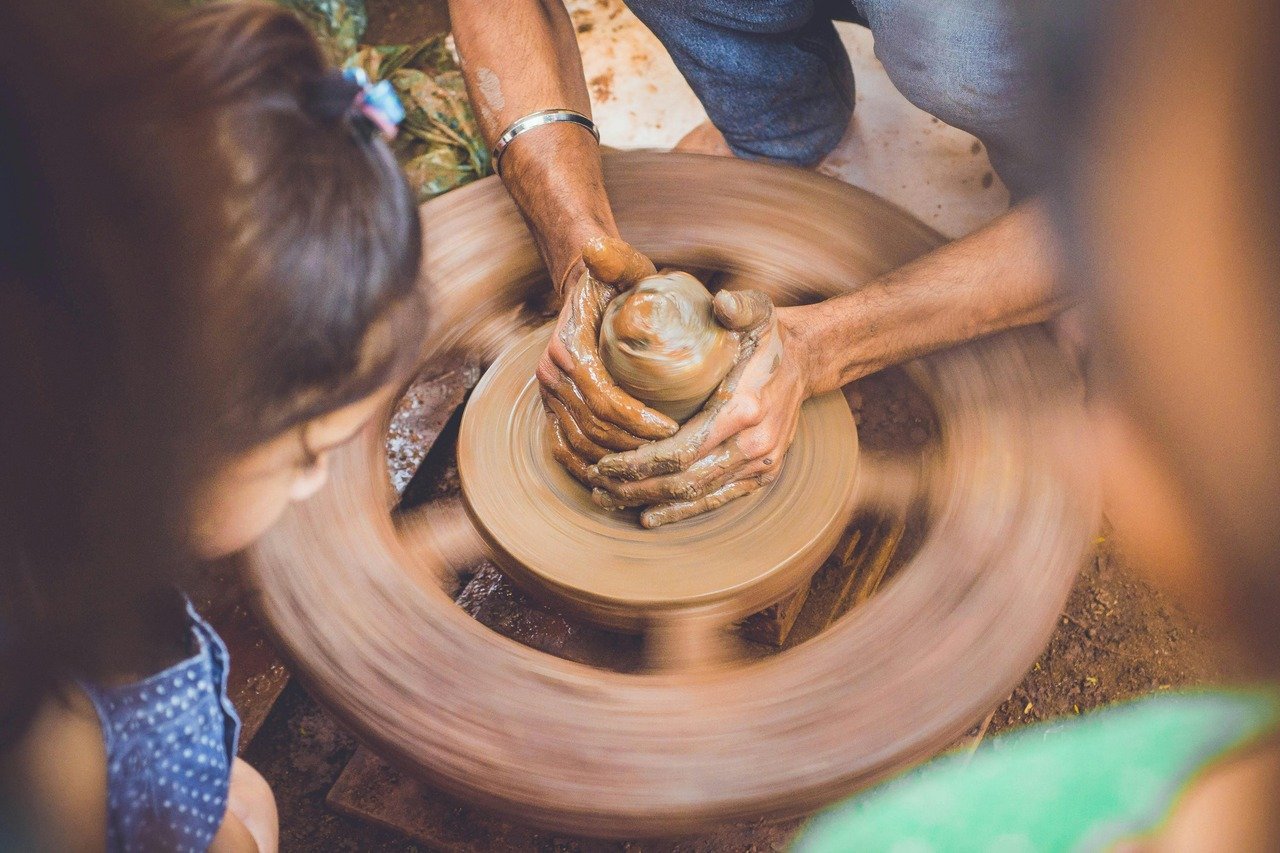
How do you manage ML experiments... Answer is MLFlow
MLflow is an open-source platform developed by Databricks to help manage the end-to-end machine learning lifecycle.
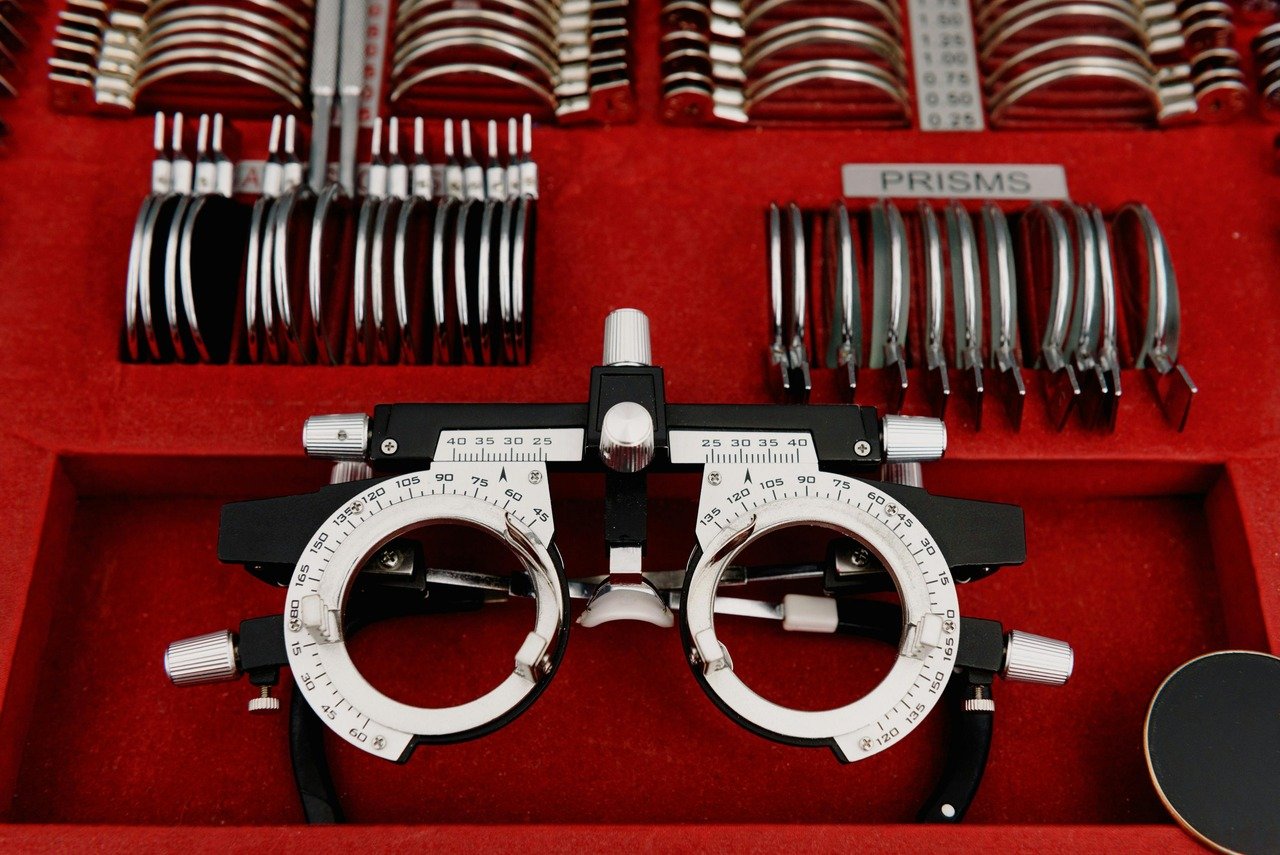
Brute Force Technique: Understanding and Implementing in JavaScript
Brute Force Technique: Understanding and Implementing in JavaScript